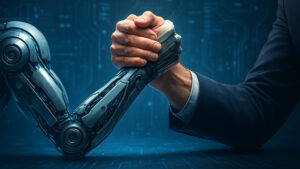
Why AI Is Not the Threat to Research Integrity — But Misuse Is
A recent article in Quirk’s rightly warned of a troubling trend: the potential for unscrupulous researchers to exploit artificial intelligence to fabricate study participants or even entire datasets. These concerns are valid — and they point to a deeper issue that long predates AI: the misuse of tools in the pursuit of outcomes that ignore integrity.
As someone who uses AI regularly in structured, transparent ways to enhance research and analysis, I believe it’s time to expand the conversation. AI isn’t the threat. It’s a tool — and like all tools, it reflects the intent of its user. What we need is not less AI, but better frameworks for using it ethically.
What AI Won’t Do for You
To clarify what AI is — and isn’t — let’s be specific. Here are examples of what models like ChatGPT will not do:
- Impersonate individuals or organizations
- Generate medical, legal, or financial claims without disclaimers
- Create false credentials, certifications, or survey data
- Provide or simulate identifiable personal data
- Evade platform content policies when prompted for unethical output
These are not theoretical guardrails. They are enforced in real time, with refusal messages that appear whenever a user attempts to cross ethical boundaries. In many cases, the AI goes a step further—explaining why the request is inappropriate.
Which brings us to the central issue: responsibility. Tools don’t have intent. Users do.
A Tale of Two Collaborations
In the past six months, I’ve led two complex research projects using AI in a collaborative, structured way. One involved a detailed question-by-question audit of research findings already produced, and the same survey being repeated this year with a defined audience. The other, a technical analysis of a specific type of specification used by an industry association. In both cases, AI augmented the human process: organizing inputs, surfacing patterns, testing hypotheses, cross-referencing regulatory documents. It was not the sole source of truth. It was a lens—one of many—through which to view the problem.
The result? Better speed, sharper insight, and yes—greater integrity. Not despite AI, but because of it.
It is simply false to suggest that using AI necessarily introduces unreliability. In fact, in a world where human bias, oversight, and fatigue remain persistent threats to data quality, AI may be our best defense.
What Is Claude?
The article references “Claude,” an LLM developed by Anthropic, as one of the tools potentially susceptible to misuse. Claude, like ChatGPT, Gemini, and LLaMA, is a large language model—a tool trained to predict and generate text based on user prompts. It doesn’t conduct research. It doesn’t verify findings. And it doesn’t invent credibility.
No AI model can.
Fabrication Is Not a Feature of AI — It’s a Human Failing
Let’s be honest: fake participants are not an invention of artificial intelligence. Fabrication in research has existed as long as research itself. From made-up survey responses to selectively omitted data points, the temptation to “massage the numbers” is a temptation rooted in human behavior—not in algorithms.
Researchers are people. And people can lie. Have we forgotten that simple logic?
In fact, many high-profile cases of research fraud over the years occurred without the use of any AI at all.¹ The tools involved? Word processors, Excel sheets, and, most often, a desire to serve a particular narrative at the expense of truth.
AI hasn’t introduced fabrication. If anything, it has spotlighted it—by raising questions about verification, auditability, and ethics that some corners of the research world have long ignored.
Misplaced Fears, Missed Opportunities
There’s an old expression in software: garbage in, garbage out. AI has not changed this principle. It has only raised the stakes. But the risk of misuse is not unique to AI. It applies to Excel. To PowerPoint. To market research firms with lax methodology. If a user wants to manipulate, the tool doesn’t matter. What matters is the culture, training, and ethics surrounding the tool.
What worries me more than fake participants is the very real possibility that fear of AI will prevent good researchers from using one of the most promising advancements in analytical thinking in a generation.
Where We Go from Here
Yes, let’s have a conversation about research integrity. But let’s ground that conversation in experience, not speculation. Let’s talk about disclosure, audit trails, and validation techniques—with or without AI. Let’s build stronger processes, not blame sharper tools.
In a phone research project a couple of years ago with an industrial designer, the conversation led to a discussion that brought out the fact that he owned a Tesla. Forgetting for a moment the disruption going on around Tesla today, during that interview, I asked the designer if he had to pick my client’s product or the Tesla, which would he chose? Without hesitating, he said my client’s product: a bidet. When we reported back to the client, the news spread like wildfire through the company. People didn’t believe it. Fortunately, we had the recording.
Today, that recording could be “faked.” Today, that interview can be invented. But what good would that do to the outcome of the client’s objectives? What good would faking results do as we pursue truth, which is the ultimate determining factor is achieving our strategic objectives?
In Call Sign Chaos, Jim Mattis wrote: “Digital technologies can falsely encourage remote staffs to believe they possess a God’s-eye view of combat. Digital technologies do not dissipate confusion; the fog of war can actually thicken when misinformation is instantly amplified.”
AI has the promise of making the fog so thick, we lose sight of ourselves as well.
AI isn’t the problem. Misuse is. And the best way to combat misuse is not to avoid the tool, but to learn how to use it wisely.
The real wake-up call? It isn’t about artificial intelligence. It’s about what we, as researchers and marketers, are willing to claim as knowledge—and how we ensure it earns that name.
There’s nothing new under the sun—not even the temptation to misuse a tool. The real test isn’t whether AI can be trusted. It’s whether we can trust ourselves.
Let us know what you think.
By Jim Nowakowski, President
Interline Creative Group, Inc. and Accountability Information Management, Inc.
_______________________________________________
¹ Historical Examples of Fabricated Studies include:
- Andrew Wakefield and the MMR Vaccine Scandal (1998) — One of the most infamous cases of research fraud in modern history. Wakefield published a study in The Lancet claiming a link between the MMR vaccine and autism. The study used falsified data, lacked ethical oversight, and was later retracted. No AI was involved.
- Diederik Stapel, Social Psychology Fraud (2011) — A Dutch social psychologist who fabricated data for at least 30 published papers. His research on human behavior and social dynamics — widely cited at the time — turned out to be largely invented. Again, no AI. Just spreadsheets, academic ambition, and a stunning lack of oversight.
- Hwang Woo-suk, Cloning Research (2005) — A South Korean researcher claimed to have cloned human embryos and extracted stem cells. His work made headlines globally, until investigations revealed he had fabricated significant parts of the data. The scientific community was rocked by the realization that such deception could pass peer review.